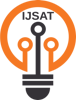
International Journal on Science and Technology
E-ISSN: 2229-7677
•
Impact Factor: 9.88
A Widely Indexed Open Access Peer Reviewed Multidisciplinary Bi-monthly Scholarly International Journal
Plagiarism is checked by the leading plagiarism checker
Call for Paper
Volume 16 Issue 2
2025
Indexing Partners

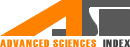
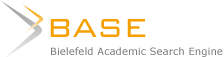

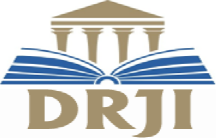

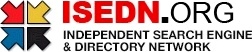

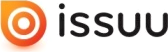

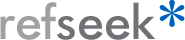
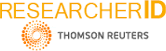
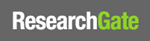
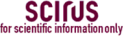
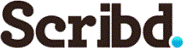

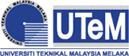
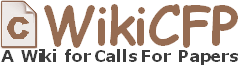
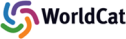
Exploring AI/ML Techniques for Deepfake Detection: A Comprehensive Review
Author(s) | Dr.Sofiya Mujawar, Harshal Madhukar Kumavat, Yash Sanjay Parihar, Kuldip Sunil Kate, Atharva Prashant Thakare |
---|---|
Country | India |
Abstract | The literature review on AI/ ML- grounded deepfake discovery delves into the evolving geography of ways designed to identify and alleviate the pitfalls posed by deepfake media. Deepfakes, which influence advanced AI to produce largely realistic fake vids and images, have raised significant enterprises regarding sequestration, security, and the integrity of digital content. The review totally categorizes discovery styles into deep literacy- grounded ways, classical machine learning approaches, statistical styles, and blockchain- grounded results. Deep literacy ways, particularly those employing Generative Adversarial Networks ( GANs), have surfaced as the most effective in detecting deepfakes. These styles use expansive datasets and sophisticated neural network infrastructures to descry subtle inconsistencies and vestiges that are reflective of manipulation. Classical machine literacy styles, while generally less effective than deep literacy, remain important for point birth and original discovery stages. The review underscores the critical part of different datasets in training and assessing discovery algorithms. Datasets that encompass a wide array of deepfake exemplifications, including colorful manipulation types and quality situations, are pivotal for developing robust discovery systems. Performance criteria similar as delicacy, perfection, recall, and F1- score are employed to measure the effectiveness of these algorithms |
Keywords | Convolutional neural networks, DeepFake, Face2Face, fake face detection, fake face image forensics, multi-channel constrained convolution, transfer learning, video or image manipulation, digital media forensics. |
Field | Computer > Artificial Intelligence / Simulation / Virtual Reality |
Published In | Volume 16, Issue 2, April-June 2025 |
Published On | 2025-04-18 |
Cite This | Exploring AI/ML Techniques for Deepfake Detection: A Comprehensive Review - Dr.Sofiya Mujawar, Harshal Madhukar Kumavat, Yash Sanjay Parihar, Kuldip Sunil Kate, Atharva Prashant Thakare - IJSAT Volume 16, Issue 2, April-June 2025. DOI 10.71097/IJSAT.v16.i2.3843 |
DOI | https://doi.org/10.71097/IJSAT.v16.i2.3843 |
Short DOI | https://doi.org/g9gdtd |
Share this

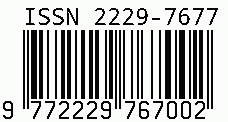
CrossRef DOI is assigned to each research paper published in our journal.
IJSAT DOI prefix is
10.71097/IJSAT
Downloads
All research papers published on this website are licensed under Creative Commons Attribution-ShareAlike 4.0 International License, and all rights belong to their respective authors/researchers.
