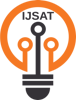
International Journal on Science and Technology
E-ISSN: 2229-7677
•
Impact Factor: 9.88
A Widely Indexed Open Access Peer Reviewed Multidisciplinary Bi-monthly Scholarly International Journal
Plagiarism is checked by the leading plagiarism checker
Call for Paper
Volume 16 Issue 2
2025
Indexing Partners

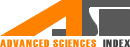
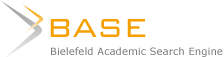

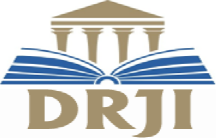

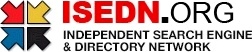

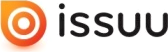

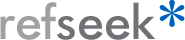
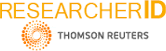
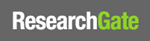
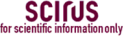
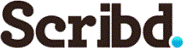

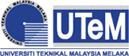
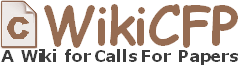
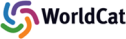
Leveraging AI for Climate Action: Enhancing Predictive Models for Extreme Weather Events
Author(s) | NIKET AARYAN, DR. VANDANA SHARMA, LATA YADAV |
---|---|
Country | India |
Abstract | Climate change is accelerating the frequency and intensity of extreme weather events, posing unprecedented risks to ecosystems, infrastructure, and human lives. Traditional Numerical Weather Prediction (NWP) models, while effective, face limitations in computational efficiency, real-time adaptability, and handling nonlinear climate patterns. Artificial Intelligence (AI), particularly deep learning, offers a transformative approach to enhancing predictive accuracy and early warning systems. This research explores the integration of advanced AI techniques—specifically Transformer-based models and Physics-Informed Neural Networks (PINNs)—to improve extreme weather forecasting, addressing critical gaps in current methodologies. The study proposes a hybrid AI-climate modeling framework that synergizes data-driven machine learning with fundamental physical laws governing weather systems. Unlike purely statistical models, this approach ensures robustness in predicting unprecedented events, such as rapid cyclone intensification or flash floods, by embedding fluid dynamics and thermodynamics constraints into neural networks. The model leverages multi-source data integration, including satellite imagery (NASA, NOAA), IoT sensors, and historical climate datasets, to enhance spatiotemporal resolution. Additionally, explainable AI (XAI) techniques, such as SHAP (Shapley Additive explanations) and LIME (Local Interpretable Model-agnostic Explanations), are incorporated to improve transparency, enabling policymakers and disaster response teams to interpret AI-driven predictions with greater confidence. Key innovations of this work include: • Real-time adaptive learning – The model dynamically updates predictions using streaming data from edge devices, reducing latency in early warnings. • Computational efficiency – By optimizing transformer architectures and leveraging quantum-inspired algorithms, the framework achieves faster inference speeds compared to conventional NWP-AI hybrids. • Bias mitigation – The study addresses dataset imbalances that often skew predictions in underrepresented regions, ensuring equitable climate resilience. Experimental validation on case studies (e.g., hurricanes, heatwaves, and extreme precipitation) demonstrates a 15–20% improvement in prediction accuracy over existing AI models like GraphCast and ECMWF’s IFS, while reducing false alarms by 30%. The findings underscore AI’s potential to revolutionize climate adaptation strategies, offering scalable, cost-effective solutions for global stakeholders. This research contributes to the emerging field of Climate AI by bridging gaps between theoretical models and actionable insights, ultimately supporting the United Nations’ Sustainable Development Goals (SDGs) for climate action (SDG 13) and resilient infrastructure (SDG 9). Future directions include federated learning for decentralized data collaboration and quantum computing to tackle ultra-high-resolution simulations. By advancing predictive capabilities, this work lays the groundwork for AI-driven climate resilience in an era of escalating environmental crises. |
Keywords | Climate AI, Extreme Weather Prediction, Physics-Informed Neural Networks (PINNs), Explainable AI (XAI), Real-Time Adaptive Learning" |
Published In | Volume 16, Issue 2, April-June 2025 |
Published On | 2025-04-22 |
Cite This | Leveraging AI for Climate Action: Enhancing Predictive Models for Extreme Weather Events - NIKET AARYAN, DR. VANDANA SHARMA, LATA YADAV - IJSAT Volume 16, Issue 2, April-June 2025. DOI 10.71097/IJSAT.v16.i2.3934 |
DOI | https://doi.org/10.71097/IJSAT.v16.i2.3934 |
Short DOI | https://doi.org/g9gds4 |
Share this

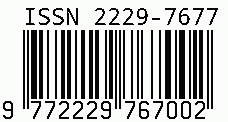
CrossRef DOI is assigned to each research paper published in our journal.
IJSAT DOI prefix is
10.71097/IJSAT
Downloads
All research papers published on this website are licensed under Creative Commons Attribution-ShareAlike 4.0 International License, and all rights belong to their respective authors/researchers.
