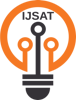
International Journal on Science and Technology
E-ISSN: 2229-7677
•
Impact Factor: 9.88
A Widely Indexed Open Access Peer Reviewed Multidisciplinary Bi-monthly Scholarly International Journal
Plagiarism is checked by the leading plagiarism checker
Call for Paper
Volume 16 Issue 2
April-June 2025
Indexing Partners

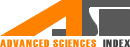
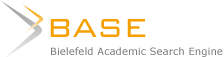

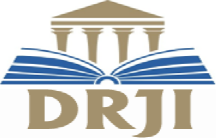

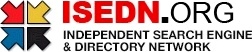

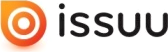

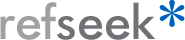
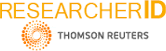
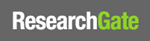
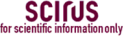
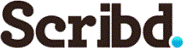

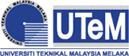
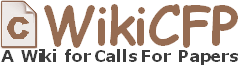
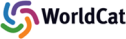
AI-Enhanced Cyberbullying Detection in Encrypted Social Media: A Privacy-Preserving Federated Learning Approach
Author(s) | Ashwin Sharma, Deepak Kejriwal, Anshul Goel |
---|---|
Country | India |
Abstract | Users benefit from better privacy because end-to-end encryption makes social media messages readable only to their sender and receiver. The new security measures make it harder to find cyberbullying and other harmful online activities. Content moderation systems based on keyword searching and content scanning stop working when users use end-to-end encryption. The urgent need for new privacy-first detection methods grows stronger because young users face more advanced online abuse daily. This research explores how AI and FL can find cyberbullying activities in encrypted messages without breaking their security. Federated Learning presents a new training method that lets individual machines handle machine learning updates locally. FL moves data processing from a server to local devices so models can refine their skills with private data without sending complete records to a central database. The suggested method uses NLP behavioral recognition and metadata analysis with AI to spot bullying signs without reading message content. The methods of differential privacy and secure aggregation help to secure data in addition to these processes. The system shows FL-based detection methods perform well without breaking privacy rules with tested research findings and practical dataset results. Our research presents three main benefits. Our approach creates a new FL system that finds cyberbullying in E2EE platforms using both user activity patterns and non-textual data. The research provides both effectiveness analysis of privacy-protecting AI systems and shows their performance against privacy sacrifices. It analyzes necessary ethical measures and compliance steps before applying these models in live setups. Our study demonstrates how AI systems that value user privacy work effectively to prevent cyberbullying and defines the direction forward for internet safety in encrypted digital worlds. Our findings create a base to make new generation content moderation systems that combine privacy safety practices. |
Keywords | Cyberbullying, Encrypted social media, End-to-end encryption, Federated learning, Privacy preservation, Artificial intelligence, AI ethics, Online harassment, Content moderation, Secure communication, Differential privacy, Secure aggregation, Behavioral analytics, Machine learning, Natural language processing, Decentralized AI, User safety, Metadata analysis, Privacy-aware detection, Encrypted communication, Social media abuse, Adversarial behavior, AI in social networks, Ethical AI, Data protection, Encrypted environments, Real-time detection, Cyber safety, User-centric AI, Anonymized data |
Field | Engineering |
Published In | Volume 15, Issue 2, April-June 2024 |
Published On | 2024-04-09 |
DOI | https://doi.org/10.71097/IJSAT.v15.i2.4011 |
Short DOI | https://doi.org/g9f7mk |
Share this

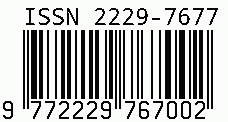
CrossRef DOI is assigned to each research paper published in our journal.
IJSAT DOI prefix is
10.71097/IJSAT
Downloads
All research papers published on this website are licensed under Creative Commons Attribution-ShareAlike 4.0 International License, and all rights belong to their respective authors/researchers.
